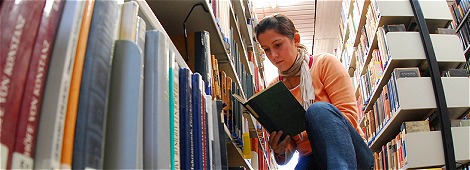
(Un)ethical NLP
Module Description
Course | Module Abbreviation | Credit Points |
---|---|---|
BA-2010[100%|75%] | CS-CL | 6 LP |
BA-2010[50%] | BS-CL | 6 LP |
BA-2010[25%] | BS-AC, BS-FL | 4 LP |
BA-2010 | AS-CL | 8 LP |
Master | SS-CL-TAC | 8 LP |
Lecturer | Katja Markert |
Module Type | Proseminar / Hauptseminar |
Language | English |
First Session | 21.10.2024 |
Time and Place | Mo, 15:15-16:45, INF 329 /SR 26 |
Commitment Period | tbd. |
Participants
Suitable for all CL Bachelor and Master students. Students from Data and Computer Science, Mathematics or Scientific Computing with Anwendungsgebiet Computational Linguistics should discuss with professor before taking the class.
Prerequisites for Participation
The types of papers are very wide ranging from basic to advanced therefore a wide range of students can take part.
- For Bachelor Students: ECL
- For MA Students: none
Assessment
- Presentation
- Discussion contributions: literature critiques, coassessment for presentations, exercises
- Second presentation or written exam, dependent on number of participants
Content
Given the large-scale development and deployment of NLP methods, and in particular but not only LLMs, a wide variety of ethical questions for NLP have arisen, including privacy and copyright issues, the representation of and impact on minorities, environmental impact of training and using LLMs, working conditions and exploitation for human annotators for LLms, and the power and political structure that can be solidified or upended.
As this can cover several seminars, we will concentrate mostly on the following topics with a focus on technical and linguistic issues (given that we are not lawyers):
- Representation bias against minorities and groups with protected characteristics in embeddings, LLMs and other NLP systems (translation, summarization etc). Methods to test for and mitigate such bias. An example paper is here
- Real world impact of such biases with regards to allocational harms as well as system performance for users of different social groups. An example paper is here
- NLP and culture, in particular whether NLP should be multicultural and culture-aware and what this means for NLP algorithm development and NLP alignment methods. We are in particular looking at the impact of culture on human preferences collection. An example paper is here
- Disadvantages of alignment in LLMs, including the problem of excess safety as well as impact on tasks such as generating compelling narratives. An example paper is here .
Agenda
Date | Session | Materials |