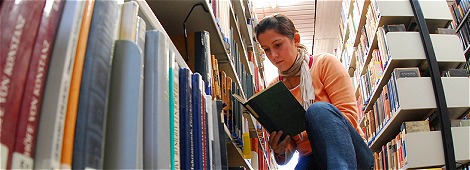
Imitation Learning
Module Description
Course | Module Abbreviation | Credit Points |
---|---|---|
BA-2010 | AS-CL | 8 LP |
Master | SS-CL, SS-TAC | 8 LP |
Lecturer | Artem Sokolov |
Module Type | |
Language | English |
First Session | 08.10.2018-19.10.2018 |
Time and Place | daily, 10:00-16:00, INF 205 / SR 11 |
Commitment Deadline | 18.10.2018 |
Prerequisite for Participation
Assessment
Module Content
This module provides an introduction into theory and practice of learning from demonstrations with a focus on natural language processing use-cases. Closely related to structured prediction and reinforcement learning, imitation learning is particularly suited for sequence prediction tasks, where often good success metrics or intermediate rewards are hard to define, while in the same time it is easy to provide demonstrations of correct behavior. After taking this module you will be able to formulate imitation learning problems, understand deficiencies of some straight-forward approaches to it, map structured prediction tasks to imitation learning, and solve them using deep learning techniques.
Module Overview
Agenda
Datum | Sitzung | Zusätzliche Materialien/Kommentare |
8.10. | 1. Introduction 2. Potential Presentations & Projects | Videos: ALVINN Super Tux Mario Speech Synthesis |
9.10. | Introduction (contd.) 3. Online learning | |
10.10. | 4. Reinforcement Learning 5. Max-Margin Structured Prediction | code exercise |
11.10. | 6. Searn (by Julia Kreutzer) 7. Behavioral Cloning | (updated) |
12.10. | (no morning session) 8. DAgger (by Dennis Aumiller) | |
15.10. | 9. Learning to Search 10. Inverse RL | (for Max-Margin IRL see lecture 5) |
16.10. | 10. AggreVaTe(D) project spot-lights: MaxIRL (by Philipp Wiesenbach, Marvin Koss, Michael Staniek) | reading group (paper1, paper2) |
17.10. | (no sessions on Wed) | |
18.10. | 12. LOLS (by Leo Born) 13. SeaRNN (by Maximilian Bacher) | |
19.10. | (no sessions on Fri) |
Literature