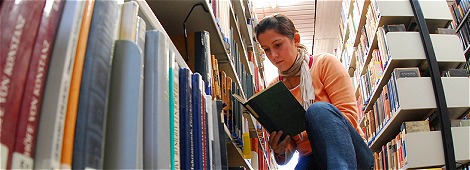
Modelling Explicit Connectives Using Span-level Attention
Abstract
Traditional approaches to discourse have shown the importance of explicit connectives(for ex, words like "since", "because" or clauses like "not only...but also", "if so") in providing coherence to a text. However in the current landscape of generating coherent text with LLMs, effectiveness of most models rely heavily on word-level attention mechanism. This rarely makes us question how such attention mechanism uses information at a discourse level. For instance, whether discourse level cues like explicit connectives are still important for producing such coherent texts. This work aims to tackle this question by looking at span level(beyond word level) attention. Our hypothesis is that such span level information exhibit tree like structures where the explicit connectives are the most important nodes. Such structural behavior is akin to what in linguistic literature is called "Lexicalized Tree Adjoining Grammar for discourse (D-LTAG)". We propose a neural shallow discourse parsing model that uses span level attention and examine their attention weights to verify our hypothesis.