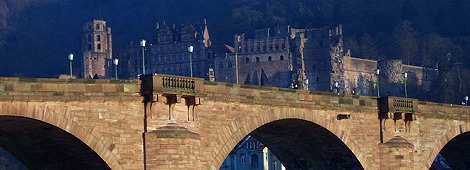
Section Classification on German Doctor’s Letters using Few-shot Learning and Prompting
Abstract
A vast amount of clinical data is still stored in unstructured text. Automatic extraction of medical information from these data poses several challenges: high costs of required clinical expertise, restricted computational resources, strict privacy regulations, and limited interpretability of model predictions. Recent domain adaptation and prompting methods using lightweight masked language models showed promising results with minimal training data and allow for application of well-established interpretability methods. We are first to present a systematic evaluation of advanced domain adaptation and prompting methods in a low-resource medical domain task, performing multi-class section classification on German doctor’s letters. We evaluate a variety of models, model sizes, (further-pre)training and task settings, and conduct extensive class-wise evaluations supported by Shapley values to validate the quality of small-scale training data, and to ensure the interpretability of model predictions. We show that in few-shot learning scenarios, a lightweight, domain-adapted pretrained language model, prompted with just 20 shots per section class, outperforms a traditional classification model, by increasing accuracy from 48.6% to 79.1%. By using Shapley values for model selection and training data optimization we could further increase accuracy up to 84.3%. Our analyses further reveal that pretraining of masked language models on general language data is important to support successful domain-transfer to medical language, so that further-pretraining of general-language models on domain-specific documents can outperform models pretrained on domain-specific data only. Our detailed experimental evaluations show that i) by application of prompting methods based on ii) general-language pre-trained masked language models combined with further-pretraining on medical domain data can achieve iii) significant improvements in accuracy beyond traditional models with minimal training data, and iv) further performance improvements and interpretability of results can be achieved, using advanced interpretability methods such as Shapley values. Our findings highlight the feasibility of deploying powerful machine learning methods in clinical settings and can serve as a process-oriented guideline for clinical information extraction projects working with low-resource languages.