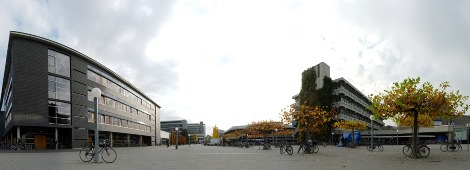
Medical information extraction using local large language models
Abstract
Medication information is crucial for clinical routine and research. However much of it is stored in unstructured text, requiring time-intensive and error-prone manual information extraction. Recent advances in NLP have demonstrated the potential of pre-trained generative language models and parameter-efficient fine-tuning methods to automate this process.
In our study we evaluate local generative large language models (LLMs) for end-to-end extraction of medication information, combining named entity recognition and relation extraction. To address the challenge of ensuring consistent structured output from generative models, we propose an automatic feedback pipeline to identify critical predictions for automated evaluation.
We experimented with two open-source models of different sizes -- one general, one domain-specific -- in zero-shot and supervised fine-tuning scenarios on an English and a German clinical corpus. While the domain-specific model struggled to generate reliable structured outputs, the fine-tuned open-source general models outperformed SOTA performance on complex relation classes, such as adverse drug events and medication reason. Moreover, a new SOTA baseline was established on the German data set. Leveraging interpretability methods, we improved prediction transparency to support physicians in informed decision-making.
Our findings suggest that generative LLMs outperform SOTA methods for medication information extraction, delivering high performance with limited time and IT resources in a clinical setup.