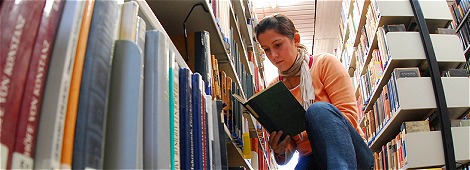
Multimodale Semantik
Kursbeschreibung
Studiengang | Modulkürzel | Leistungs- bewertung |
---|---|---|
BA-2010 | AS-CL | 8 LP |
NBA | AS-CL | 8 LP |
Master | SS-CL, SS-TAC | 8 LP |
Magister | - | - |
Dozenten/-innen | Gerhard Kremer |
Veranstaltungsart | Hauptseminar |
Erster Termin | 22.04.2013 |
Zeit und Ort | Mo, 16:15–17:45, INF 327 / SR 2 (SR) |
Commitment-Frist | 20.05.–13.07.2013 |
Teilnahmevoraussetzungen
- Vertrautheit mit Statistik und mathematischen Grundlagen der Computerlinguistik
- Voranmeldung bis 19.04. per E-Mail beim Dozenten
Leistungsnachweis
- Aktive Teilnahme an den Diskussionen
- Referat und schriftliche Ausarbeitung
Inhalt
Datengetriebene (vor allem distributionelle) Modelle der Wortbedeutung in der Computerlinguistik beruhten bis vor wenigen Jahren ausschließlich auf großen Textkorpora. Es gibt jedoch gute Gründe, sich nicht alleine auf Text als Wissensquelle zu stützen. Diese kommen sowohl von der kognitiven Seite (Menschen lernen Wortbedeutung selten aus Text allein, sondern Wahrnehmung spielt auch eine wichtige Rolle) als auch von der linguistischen Seite (offensichtliche Informationen wie "Gras ist grün" sind in Korpora schwer zu finden, da sie nicht informativ wären).
Eine neue Forschungsrichtung in der Computerlinguistik hat daher zum Ziel, für die Modellierung von Wortbedeutung auch Information anderer Modalitäten mit einzubeziehen, zum Beispiel menschliches Wissen über Konzept-Merkmale, Assoziationen oder visuelle Information.
Wir werden uns im Seminar mit der Erstellung, dem Umgang und Anwendungen von verschiedenen Bedeutungsrepräsentationen beschäftigen und den Methoden aus der Computerlinguistik, die dabei angewendet werden.
Kursübersicht
Seminarplan
Datum | Sitzung | Materialien |
22.04. | Einführung | Einf.pdf |
29.04. | Referatsvergabe, Bewertungskriterien | |
6.05. | McRae, Cree, Seidenberg & McNorgan (2005): Semantic Feature Production Norms for a Large Set of Living and Nonliving Things |
|
13.05. | [Referat: Spaar] ... [Schriftwerk: Kostyra] Turney & Pantel (2010): From Frequency to Meaning: Vector Space Models of Semantics (Schwerpunkt: Abschn. 1 bis inkl. 4.4 und Abschn. 8 & 9) |
|
Pfingstmontag | ||
entfällt | ||
3.06. | [Referat: Glockner] ... [Schriftwerk: Haider] Andrews, Vigliocco & Vinson (2009): Integrating Experiential and Distributional Data to Learn Semantic Representations (ohne quantitative Analysen, d.h. S. 479-484) |
|
10.06. | [Referat: Haider] ... Silberer & Lapata (2012): Grounded Models of Semantic Representation |
|
17.06. | [Referat: Haas] ... [Schriftwerk: Glockner] Johns & Jones (2011): Construction in Semantic Memory: Generating Perceptual Representations With Global Lexical Similarity |
|
24.06. | [Referat: Kostyra] ... [Schriftwerk: Haas] Leong & Mihalcea (2011): Measuring the Semantic Relatedness Between Words and Images |
|
1.07. | [Referat: Pilkevich] ... [Schriftwerk: Ramsl] Bruni, Boleda, Baroni & Tran (2012): Distributional Semantics in Technicolor |
|
8.07. | [Referat: Feuchtmüller] ... [Schriftwerk: Spaar] Feng & Lapata (2010): Visual Information in Semantic Representation |
|
15.07. | ... [Schriftwerk: Pilkevich] Bergsma & Goebel (2011): Using Visual Information to Predict Lexical Preference |
|
22.07. | [Referat: Ramsl] ... [Schriftwerk: Feuchtmüller] Speriosou, Brown, Moon, Baldridge & Erk (2010): Connecting Language and Geography With Region-Topic Models |
Literatur
Baroni & Lenci (2010): Distributional Memory: A General Framework for Corpus-Based Semantics
Andrews, Vigliocco & Vinson (2009): Integrating Experiential and Distributional Data to Learn Semantic Representations
Bruni, Tran & Baroni (2011): Distributional Semantics From Text and Images
Blei, Ng & Jordan (2003): Latent Dirichlet Allocation
Griffiths, Steyvers, Tenenbaum (2007): Topics in Semantic Representation
Steyvers & Griffiths (2007): Probabilistic Topic Models
Yang, Jiang, Hauptmann & Ngo (2007): Evaluating Bag-of-Visual-Words Representations in Scene Classification
Wing & Baldridge (2011): Simple Supervised Document Geolocation with Geodesic Grids